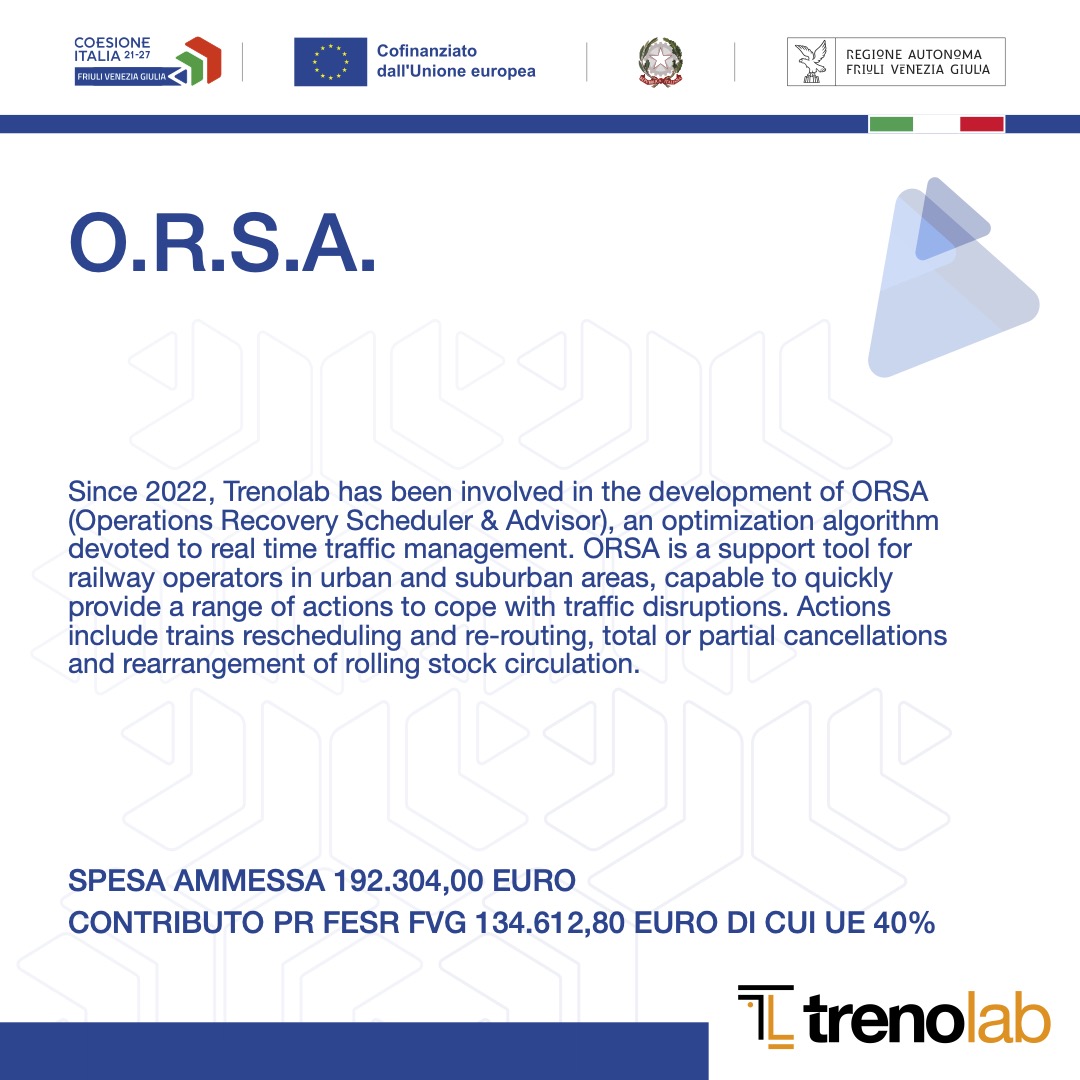
A real laboratory
trenolab works to remain a real laboratory of railway operations, in which the processes and tools are relentlessly improved. Thus applied research is part of our everyday day life.
In it, we combine the skills learned in the years of PhD and research contracts with the experience gained working on real projects to focus our activity on the challenges that railways face.
We believe that sharing the results of research helps progress in railways, and thus are glad to present them in journals and conferences. Additionally, we support sponsor conferences and other events.
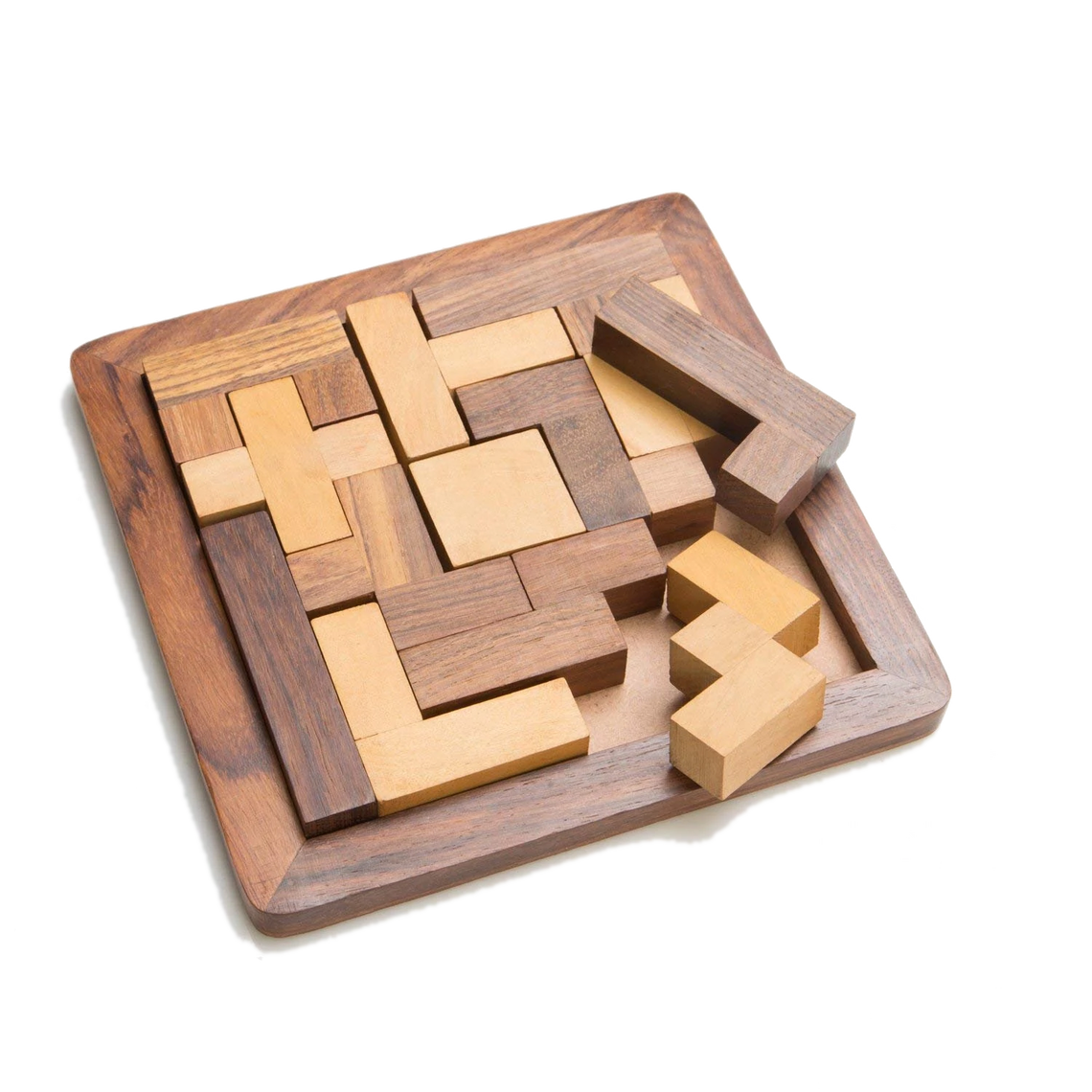
Automatic timetabling
trenolab is currently developing and updating a tool for automatic timetabling, to be included in the functionalities offered by the TRENOplus module.
The ATMO (Automatic Timetabler with Multiple Objectives) algorithmic framework has been developed to effectively produce Pareto-optimal sets of timetables optimised according to KPIs as, for instance, the travel time, the energy consumption or the number of scheduled trains. ATMO exploits an original Multi-Objective Ant Colony Optimisation algorithm to perform a quick exploration of the solution space, whose results (timetables) are then further refined by an exact Mixed Integer Linear Programming formulation.
As far as we know, the integration between these two types of algorithms is a novel contribution to the field of the solution approaches for the Train Timetabling Problem. The core of the ATMO framework has been developed within the research project “Tools for mathematical optimisation of strategic railway timetable models” funded by Jernbanedirektoratet (Norway) in 2021, and it’s currently under constant updating.
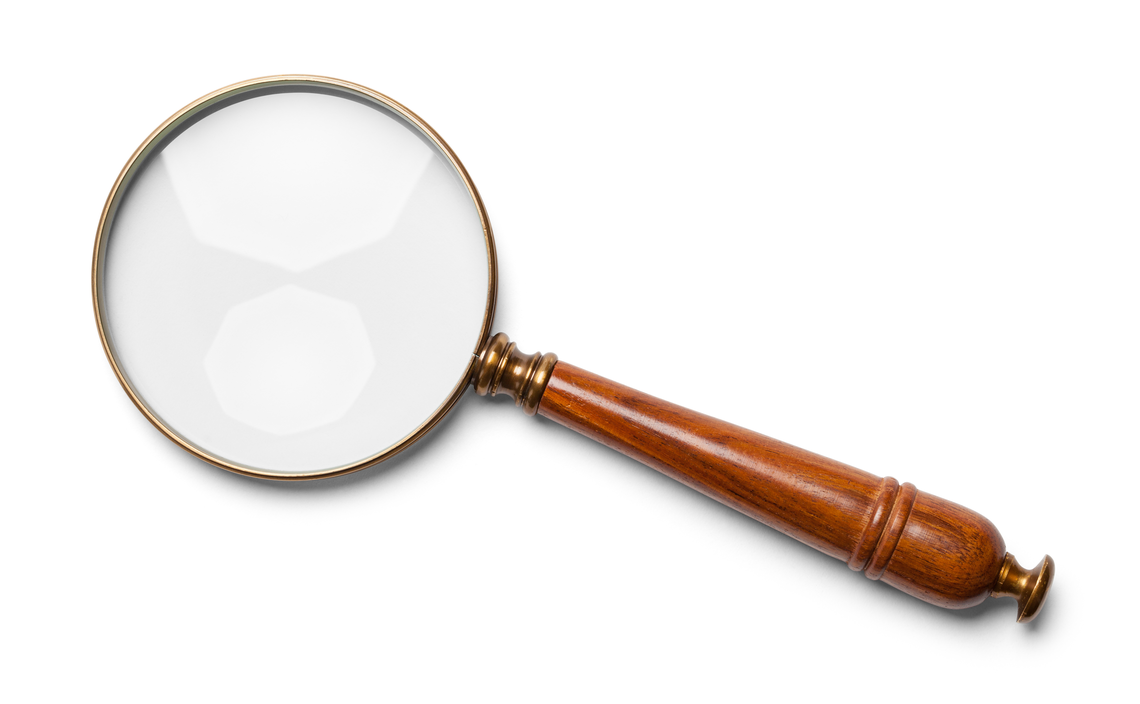
Analysis of operational data
Understanding how real train operate is a key basis to develop accurate simulations. At trenolab, we receive and analyse data logged on the infrastructure as well as on board of trains.
On-board collected data such as GPS or PTMR are used to fine-tune the behaviour of virtual drivers, while track circuit data are used as input for delays.
Additionally, passenger data coming from AFC systems are used to estimate dynamically and with highest accuracy the number of passengers that would board on each train as function of the time of the day, its delays and the delay of the previous services.
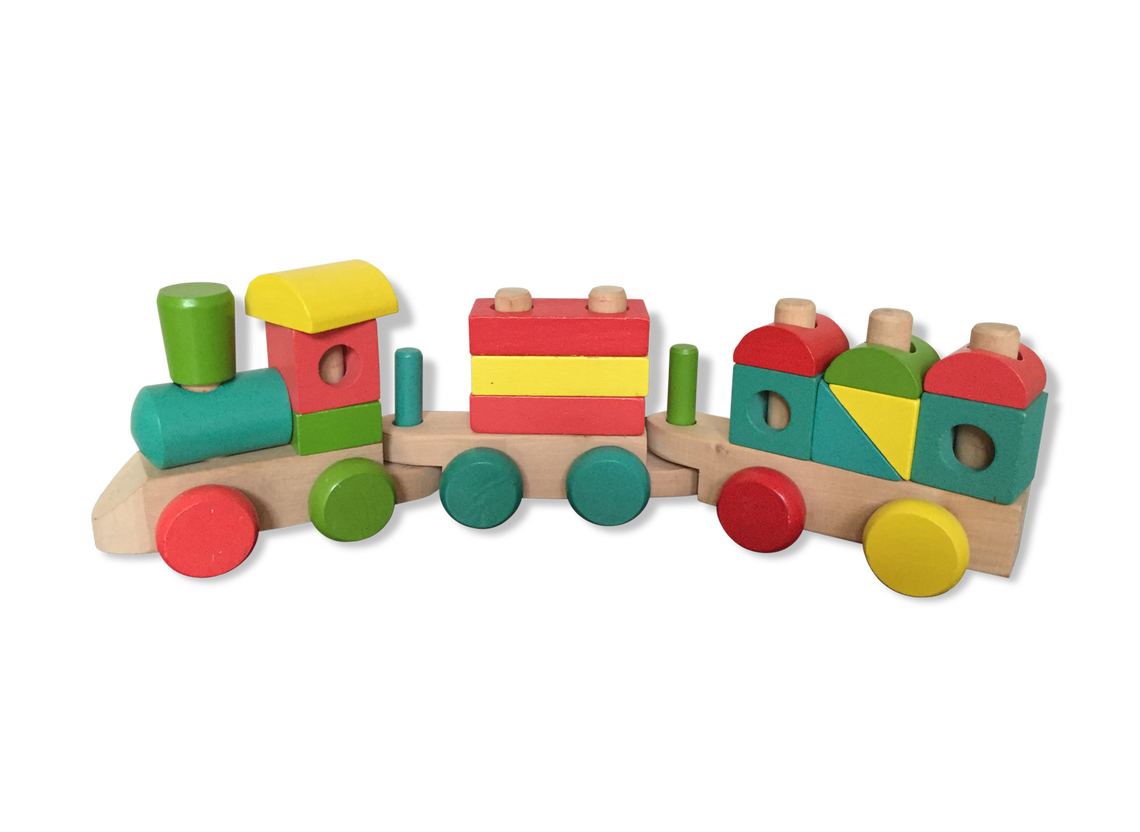
Microscopic simulation
trenolab leads the way towards a microscopic simulation that is accurate and fast enough to become part of the normal planning process.
To reach this ambitious goal, we work in parallel to: reduce the computation times, streamline the workflow for model preparation and calibration, and increase the accuracy in the representation of humean factors (as described in the paragraph on Analysis of operational data).
One key example of this continuous and successful process is the capability of trenissimo to split the simulation load not only among all computers connected to a local network or a cloud, reducing the simulation time to justa fraction of other tools.
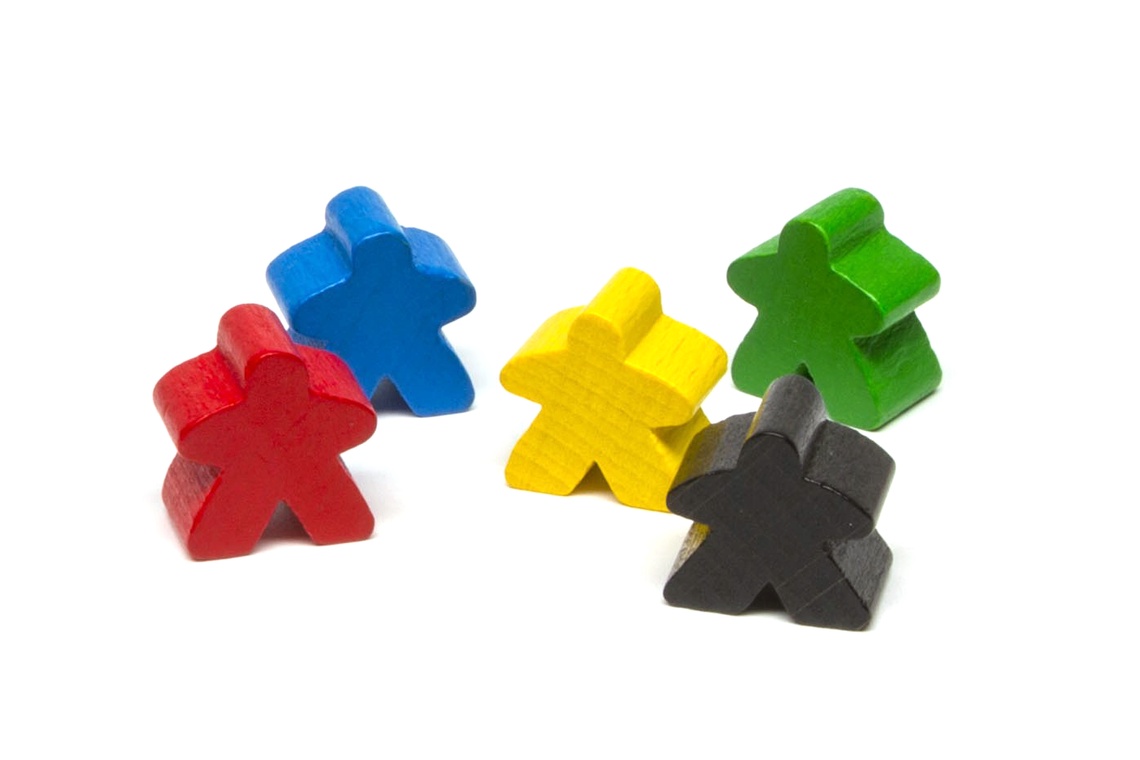
Crew rostering
The TRENOcrew module takes advantage of a novel algorithm for solving the rail Crew Scheduling Problem (CSP). The latter consists in defining a minimal set of daily train crew duties which cover a given set of train services, and can be modelled as an extension of a Capacitated Multi-Depot Vehicle Routing Problem.
For TRENOcrew we adopted a novel Ant Colony Optimisation meta-heuristic algorithm to effectively solve CSP instances of up to medium-large size (some hundreds of daily courses). Decomposition techniques are currently under design and development in order to tackle also large-size instances.
Moreover, further research is carried on to extend the proposed algorithm to solve the Crew Scheduling and Rostering Problem, featuring the additional task to calculate feasible rosters (sequence of daily duties assigned to the same driver over a monthly - or more - period) for the generated daily duties.